Lung Cancer Early-Diagnostic CNN
Developing an Convolutional Neural Network for Accurate and Inexpensive Lung Cancer Diagnosis

Abstract
Cancer is the second most common cause for death of Americans, only behind heart disease. In 2020, the number of new cancer cases is expected to exceed 1.8 million. Lung cancer is one of the leading causes of death; almost 25% of all cancer deaths were caused by lung cancer. Lung cancer is deadly primarily because it is difficult to diagnose and catch in the early stages. So, if it could be caught at an earlier stage, millions of lives could be saved. This experiment focuses on the application of machine learning to create a Convolutional Neural Network using Lung CT scans to exceed the current efficacy of 89%. The proposed hypothesis is that if an improved model is created, then it will diagnose cancer at a higher accuracy than the 89%. First, the appropriate dataset was found from the LIDC, a database of lung cancer images at various stages to train the model. Next, the data set was imported with the necessary packages in Python and was labeled. Then, the images were preprocessed for an efficient program run. Then, the model was created and trained with the remaining half of the dataset. Next, the efficacy of the run was noted and compared to the current threshold. It was found that a Convolutional Neural Network could be made that exceeds the current 89%. This has tremendous applications in the field of healthcare; if lung cancer is caught in early stages, then the number of deaths can be greatly minimized.
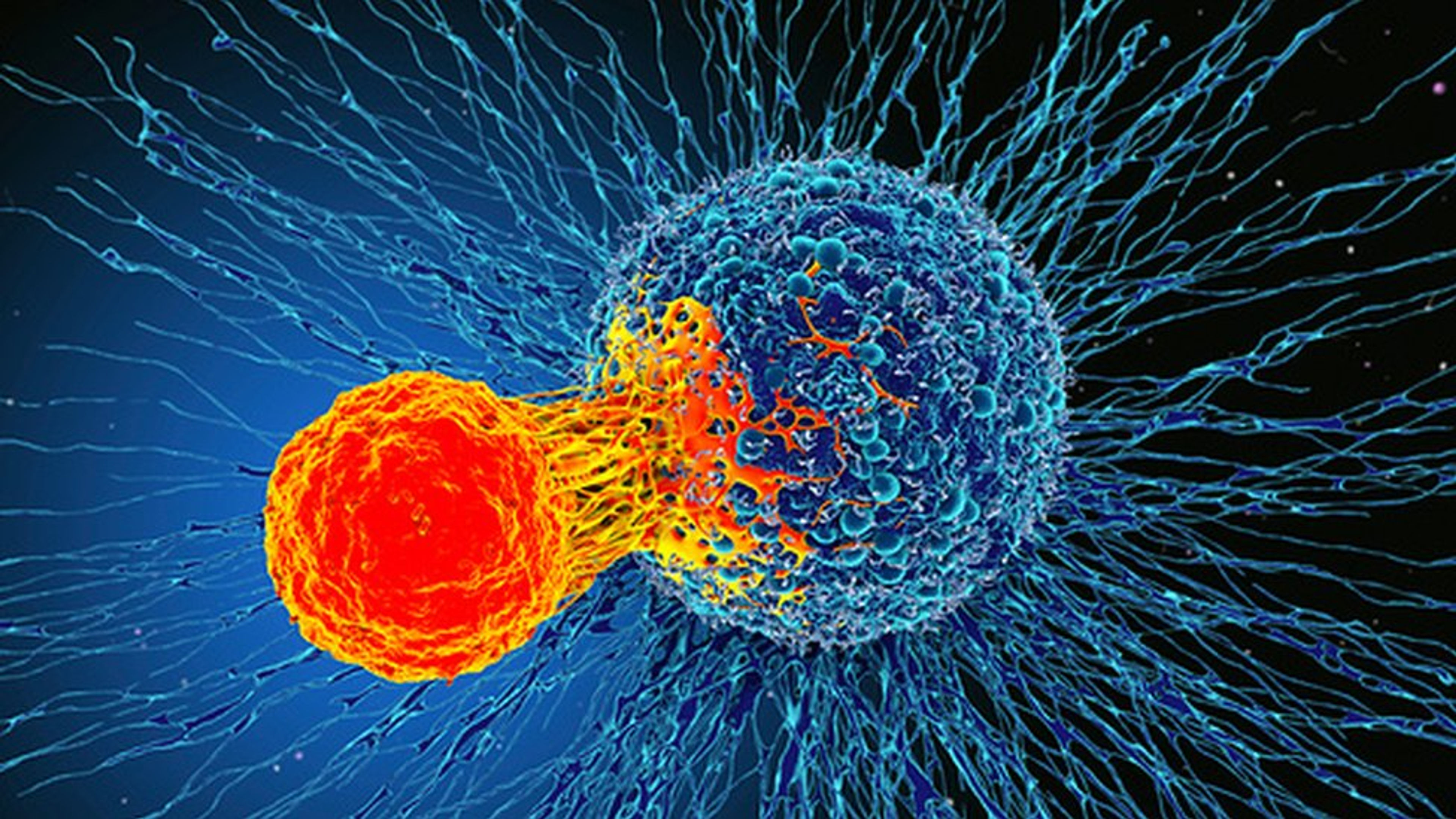
All Videos
Presentation
Comments
Please leave your comments here and in the floating discussion widget. If you have further questions, don't hesitate to reach out to the email address below. Thank you so much for taking the time to judge this project!
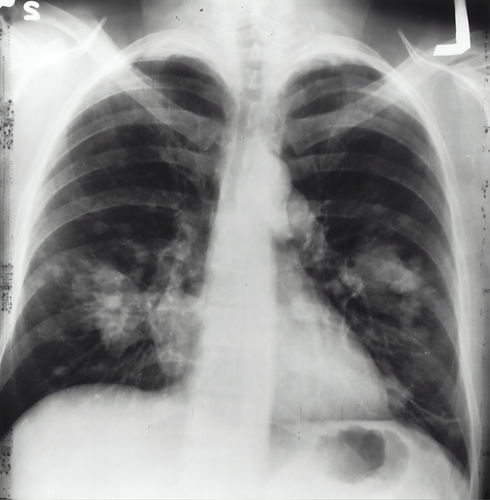